Reimagine Your Data Catalog with AI Data Assistants
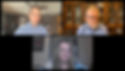
Recorded April 4, 2024
Generative AI is changing the way we learn and work. Its impact on an enterprise's data, analytics, and governance is no exception. In this webinar John Wills, the founder of Prentice Gate Advisors and renowned catalog and governance expert, will discuss and demonstrate how chat-oriented AI Data Assistants are emerging as the primary tool for achieving real growth in a data culture.
​
Moderator Christopher Johnson is the Managing Partner of Data Visuals and a former data executive with Alation, Harley-Davidson Motor Company, and Miller Brewing.
Listeners can expect to learn:
-
The definition of an AI Data Assistant
-
The role of the catalog with an AI Data Assistant
-
Value and impact for all employees, analysts, and stewards.
-
Data governance-related opportunities and impact
-
Practical advice and next steps for data & analytic leaders
​
​
Transcript
​
​
I can have a chance to respond in the chat while we, uh, while we talk. I'm, I'm outwitted by doing two things at once. So, uh, I'll just say hello verbally.
Yeah. Why don't we go ahead and get started, Samantha? Sure. Yeah. Good call. Okay, so thank you everyone for joining. Welcome to Reimagine Your Data Catalog with AI Data Assistance. Um, so I will say this is being recorded, um, and we will be sure to send the recording out after the webinar. Um, my name is Samantha Wills.
I will be moderating the questions you might have, and we will be asking those at the end of the session, um, so please feel free to, uh, enter those in the chat bar. Um, and then if there are any technical questions or any questions that I am able to answer as the webinar is happening live, I'll be in on the back end just kind of typing responses.
So, um, yeah, feel free to, um, raise any questions and we'll be sure to get them answered. So, um, I want to go ahead and introduce our moderator, Chris Johnson. He's an experienced principal advisor and technologist with 30 years in data management, architecture, and enterprise data operations. He's a former technology executive for Alation, Harley Davidson, and Miller Brewing Co.
And he has designed and guided data solutions for regional and global teams, spanning a wide array of business and technical use cases. He is also active in the broader data community, um, through his work as a contributor for
of knowledge, second edition, as well as his blog, the better data SME. Samantha. And thanks so much for having me, John. Good to see you again. As always, uh, I need to cut out the 30 year part. Cause every time I hear that it's sounds like, Oh boy, that's a long time. Also, uh, you know, Note to self just to reach out to the demo folks and say, we need to think about marketing in terms of naming conventions on some of those materials, but I'm really glad to be here.
I mean, it's going to be a hopefully a really valuable discussion for everybody. You know, AI is is taking the world by storm there. There's a lot of information coming in. Um, what we've try to do and what we hope to do today is to kind of demystify it as more of a practical application through this notion of AI assistance.
Um, and John and I have been working specifically around this and, and today we're going to kind of anchor that really around data cataloging. Um, we, we both have a passion for that and some experience in that space. Uh, so hopefully it'll be a good conversation. Um, Um, we're going to share with you some of the capabilities that we've actually started to build, so we'll do a little bit of show and tell.
But primarily, um, we will share our kind of perspective and thoughts about, you know, this whole emerging space and how you can bring it to bear to do better data management. So, John, great to see you, as I mentioned, as always. Um, maybe a quick intro for those who don't know you. Yeah, great. Thank you, Chris.
Uh, so John Wills, founder and principal of, uh, Prentice Gate Advisors, um, which is a data advisory consultancy. Um, a lot of great clients that I work with, um, mostly data leaders, but on strategy and architecture and Chris and I have known each other now for a good, good many years and work together, done podcasts together.
We've, we've done some joint writing. Uh, on various topics all around the data space. So thank you very much, Chris, for doing this. Really appreciate it. And Samantha, thank you very much for all the logistics. And first and foremost, thank you to all of our attendees. I really appreciate everyone spending some time with us.
I'm super excited to dig back into this topic. You know, so much is changing so quickly in this landscape. And I know that we did a webinar last month, but even in that short space of time, a lot's changed. It's, it's just a super exciting topic. So I'm really anxious to dig in. One logistical thing before, uh, I know Chris, we're going to dig into this.
Samantha, I did see one, someone in the chat say no video for John and Chris. So are we, are we okay on the video? Do we think we're good? I can see you guys, so. If you think we're good, we'll just press forward. I think we got a thumbs up. Yep, absolutely. Thank you everyone. very much. All right, Chris. So why don't you kick us off and let's time to dig into the topic.
Yeah, I know that you mentioned that, um, you had covered some of this specifically around AI data assistants. But for those folks who either were not able to attend your webinar or you may have not been able to look at, I think there's a recording of that for sure. So if you haven't, go back and follow up folks if you're interested in that.
But for those folks who haven't, let's just kind of quickly go over it again. What is an AI data assistant? Who needs it and why? Right, right. Great. I mean, good, you know, good context setting question. So I appreciate that. And, and for those of you who haven't figured it out already, Chris and I are going to do the, uh, podcasty discussion style, very informal.
So as Samantha said, we'd love to entertain your questions there at the end. So please feel free in the chat, but, uh, Chris, your question. So, you know, look, we, we are all very familiar with, uh, you know, gen AI and what's happening in space, um, at least at one level or another. Right. And so. No, this idea of assistance is, is really like to most of us who are careful watchers of the space, it's nothing new, but what the twist for me is for data systems is really to allow data leaders to have leverage and to uplift what their organization can provide to the enterprise, right?
So the twist with data assistant is. To provide knowledge, to provide, um, potentially access to provide analysis, to provide mentoring, instruction, guidance, all of those things, um, around the topic of enterprise data, um, for the enterprise, right? So it's, it's now a vehicle for a data leader to, you know, we all talk about data, data culture, and how do you do that and how do you get adoption?
And what does it all mean? And now we have this wonderful vehicle. It's kind of ironically, a super simple interface, right? Chat. Who would have ever thought, right? We've spent decades building very complex UIs on all kinds of applications. And we all end up again at chat, but super powerful, super easy to use.
Super, you know, zero learning curve ability for a data leader to take. Enterprise specific data knowledge and and have it be, you know, uh, the ability to have it be virally accepted. I mean, in a positive way, right? Uh, adopted throughout the enterprise, right? So a data system is the coming just to wrap that up.
It's the coming together. Of the capabilities we all know and things like chat GPT and things in the public domain where you can just ask questions, have a conversation to come in together of that with enterprise specific knowledge, right? Which is more recent and obviously directly applicable to things people need to, you know, ask questions about to get work done, bringing to coming together of those two things in a very easy to consume package.
Probably the best way I could explain it. Yeah, I think that's good. The only thing I would add to that is, um, you know, certainly, certainly provides that mechanism for executives and for leaders in the business. I think it really opens up the data world to mere mortals, right? So anybody that isn't either technically inclined, the notion of natural language being kind of the medium for how you do this, but also then Yeah.
To be able to independently just ask those questions and use this kind of technology ad hoc at will, right? Um, so without question, without question, let me add one thing to that. We're talking about catalogs specifically here. We're going to, we're going to probably range a little bit well beyond that, but, but we're We're going to focus, try to stay focused on catalog.
We all know the adoption story, right? I mean, I've worked at two major, you know, elation and Calibra, and you worked at various places as well. And we've seen lots and lots of attempts at adoption and there are success stories. There's no question, but I think it's also fair, safe to say we haven't seen the, the adoptions by the masses of cataloging, which are supposed to bring the knowledge base to mere mortals, to use your words, and we just haven't seen it and I have data leaders all the time.
Uh, ask me the question, you know, how do we achieve adoption? How do we achieve higher rates of adoption? And, you know, there being the C suites and every one of the enterprises, I know many on this call are probably feeling this pain, uh, are really demanding that data and analytics be put at the center of the universe in terms of business growth and business agility, and, um, in some cases, risk mitigation.
But, you know, it's, it's really, we're, it's a positive thing. We're moving into the hot seat, but it's also a negative thing. And it's, you know, it's a challenge to say, how do you move into the hot seat with data and analytics at the core of the enterprise? And achieve that. How do you have adoption? And, um, and so, you know, data assistance, I think, is a new opportunity for us to, uh, to achieve that.
Yeah, I agree with that. I think it's great. Great point. Um, the last piece that I'll add to that, you know, from my point of view, too, is, um, I think, well, certainly it's early. This is nascent technology. This is really disruptive. This is a trend, a big transition in terms of how people are consuming data and information.
And I think the future, you know, is is very bright here. But, um, getting a little bit more specific, what kind of information really should be built into a data system? So, you know, It's this really cool magic form. How will people want to, to develop this, this capability? Well, and I, for those of you who, you know, we're going to, you know, I know we're, we started pretty abstractly with the question.
This is going to be another somewhat abstract answer. We are going to head towards the demo. Chris is going to do a demo. Of some of the stuff we've been working on so for those of you who want to look at more tangible stuff I just want to give you that heads up But let me first, um talk about your the answer to your question chris and I want to share something.
I just polished off a um, My dude, you know many of you know, i'm a columnist on uh on tdan Uh, which is a venerable 30 year in the industry, you know, sort of newsletter. Um, I just finished my latest article. So, um, hopefully everyone can see it. It's, uh, called AI data assistance, a data leaders, force multiplier.
And, you know, when you talk about what kind of information I'm going to hit you with three things here really quick. Uh, first of all, I have this table here inside the article, which sort of says. You know, go around the damn wheel or, you know, you, you sit down and pick out your favorite categories of in the data world.
I've got governance here and stewardship and quality and just, you know, descriptive analytics and predictive and data architecture and master. Pick the domain in the data space and, and yes, right? Those are all valid targets for having a data assistant be an expert on those topics. And so you kind of have to look at your organization and say, where do you have gaps?
Where do you have holes? Where do you want to have basically I'm going to say hire a new expert You're going to hire that expert in the form of a data assistant And and on what topics right because you're going to have this you're going to tell it That its persona is an expert on these things, right?
So so I think these are all fair game and there's some introspection that needs to happen within an enterprise The other thing I want to share is that, you know, when you, when you think there, so there's some of the topic areas, but then you think about the level, you know, what is the, what is the job role you're hiring for?
Right? Again, if I can make that analogy and, and leap to a data assistant as a new employee, you're going to hire, well, what roles do you need to fill? And so this is the way I think about, uh, Uh job roles, right? You librarians and curators and you have, you know, people that perform in a role of instructor or teachers Analysts builders consultants, right?
Um, so what role are you hiring for? Right? And and so you need to ask yourself that question as well And then you have to ask okay for these topic areas for these roles What level of expertise am I hiring for and so I use the classic consultant Consulting matrix, right? So it's, it's your, your roles down the left and it's your topic areas across, you know, across the columns.
And, and then I use the numbers to indicate what level of expertise, expertise, my hiring someone out of school or one to three years of experience or five to 10 or, you know, whatever. Um, and so you really, I think when, when this is different from the last time, you know, we did a webinar and I think some of this, the thinking is really starting to crystallize.
It's thinking about hiring someone and thinking about the combination of domain expertise and the role. And then, and then what level of expertise? So I would encourage, uh, and I'll probably reinforce this point later, but I've encouraged people to, to think about this and, and, and use this analogy of hiring a human.
And being reasonable about what the job description is and what you're really targeting for the data system. So does that mean I'm going to stop sharing now, but does that mean in the future, we may end up with, um, with many different data assistants of various types within the data space? Sure.
Absolutely. Um, I don't know. Did that answer your question, Chris? Or do you have any, I'd like to see what your reaction to that is. It did, but if I distilled that down, I think the key takeaway for me is that when we start thinking about what do you put in, where do you get started, you can start with a lot of different perspectives, but it probably makes sense to not boil the ocean like within any, you know, type of enterprise or corporate project, right?
Start with a particular functional area that really needs more lift, uh, to get to drive adoption and to get that data, uh, intellect, you know, muscle moving, but then also focus on teams, right? If there's a particular team that really needs help, say you've got a new epic for the business. So choosing privilege of focus, right?
On a certain area to start a data assistant. And then to your point, You know, theoretically, and we're still working through. What does that look like? You can create a number of data assistance or just give the data assistant different perspective. So depending on my role and what function I work in, I may go in and just select that function and say, this is what's important to me, which helps to narrow it down.
If I, if I'm picking up what? Yeah, absolutely. And just a couple other thoughts on that. First of all, we all know that. Well, two things. Catalogs really are great. System of reference, right? They're primarily a reference vehicle for looking things up. Now there are some catalogs that let you do things like query and, um, look at data quality and compute data quality and.
You know, find sensitive data values and that sort of thing. So they, they stretch beyond just system of reference there. They actually start applying some analytics and intelligence to the data and allowing you to work with the data, but large, largely they're systems of reference. So when you look at those roles I laid out and you say a librarian, an instructor, a light analysis, that's where we know LLMs and gen AI is really good right now, right?
You move into the consultative and decision makers and that sort of stuff. We know Gen AI is still really, really early, right? And, and we also know there's some other more traditional machine learning and, um, sort of, uh, you know, more symbolic sort of, uh, reasoning engines that you may want to use to apply some of those other, those problem domains.
So my point is that, you know, yes, you have to be reasonable at what you can put in scope for your first data assistant. And I think, I think all these things need to be considered. The other thing I would say is I want to go back to the adoption thing because I was thinking about this analogy the other day and I, I've tried it on a few people.
I'll try it on everyone here, but for me, you know, catalogs as this great. System of reference. You know, when I went to the university, I went to, you had a 10 story library. It's even massive library, all this reference information, expertise on people, you know, in the, the author card catalog and subjects and the subject card catalog and all these floors, you know, I think this adoption problem with catalogs is a lot like you walk into that ground level of the library.
And you walk up to the librarian and they're like really smug and they don't want to wait on you. And like, they're really hard to communicate with. And like, You just don't like the librarian, right? All this information sits there, and that's what I think the UI is in a lot of catalogs, right? Like, we say, why, why aren't users throughout the enterprise adopting?
They just don't like, they just don't like the librarian, right? What we need is a nice, friendly, you know, cordial, easy to communicate with librarian. And I think that's, you know, to dumb it all down, like, that's the simple opportunity, I think. Chatbot based, Data systems kind of give us as a window into the world.
Again, that's starting at that intro level of role, right? A librarian instructor, excuse me, instructor, light analyst, and so forth. Anyway, so it says maybe that analogy works for people, but, um, yeah, it's, it's. I mean, everybody is going to kind of think about this slightly different, but to me, as we think about this and just in my experience, and I think several people on the call probably can echo this is there tends to be a gravitational pull to.
Uh, technology and to the technical aspects of data. And that always becomes a challenge for adoption. But having said that, um, I know that there's a lot of data leaders who, who've already along those lines made really significant investments in, you know, analytics and training and development, uh, as well as cataloging cataloging can, you know, can get expensive.
Um, data governance, all of these programs, they all have a footprint and a cost. And sometimes it's, it's difficult to, to, you know, really measure what the return is on that. But having, having that as a background, let's, can, can you just help us understand a little bit about how the AI data assistant either replaces or complements those programs and those investments?
Yeah, great question. Cause I, you know, I think there is a lot of, You know, fear, uncertainty and doubt, right? People make large bets, large investments. And, and, you know, the last thing any of us want to do is walk into the boss's office and say, I'm sorry, just kidding. I'm going to do something completely, completely different.
Right. This is a bit of a career limit. So, um, so yeah, it, it actually, um, I think data assistants are extremely. Uh, complimentary. Uh, if that hasn't been obvious already from my previous comments to cataloging governance and all those other data areas. Um, why do I say that? Well, like, let's again, stay focused on cataloging, um, you know, an AI data system is only going to be as good as the knowledge that contains and a really, really, um, high value, you know, Okay.
for capability that catalog provide is a very wide array of connectors to all kinds of enterprise data sources, B. I. Reporting sources, right? A vast set of sources. Those are in place. Those are doing a great job of collecting. And then, like I said, uh, some catalog platforms actually then do some of their own analysis and provide extra metadata, extra knowledge or analysis on top of that, which is which is great and can all be consumed into.
a large language model, um, for, for this experience that I've already described. So I think it's extremely complimentary. In fact, I think the capability on that side of the catalog actually becomes more important, becomes more entrenched, um, to, to the enterprise. Um, so, yeah, so I think it's extremely, extremely complimentary.
And I also add to that, like, if you look at governance and what happens with cattle, Governance in the intersection there, you always have experts and people in the central program office who need a set of tools with their own UI to go in and curate and govern and approve and all of those things. And so even the UI, which I've, I've sort of, I guess, uh, uh, maybe not talked about so, UI has great value for a certain, uh, role and a certain role type that has to continually manage these processes.
Right. Um, so I think, I think it's extremely complimentary. Good. Do you have any thoughts on that or agree? Disagree? Well, I, I want to be a good moderator, so I'm not going to say that. No, I think you nailed it. Right. And when we start thinking about that, there is going to be as, as this technology shift and this disruption moves its way into the enterprise, I think we're going to find a lot of.
Platforms and tools and processes that support them and even roles. I hate to say it will become obsolete by design, right? So I I you know that I've shared this with you before but for those on the on the call, um, I Strongly believe that we are looking at the the television to the radio world transition Uh, this is this is going to be significant, right?
This is cars to horses I think it's super early right now, but having said that The challenge for leaders is how to manage that investment. And I think what the AI data assistant brings to the table is a transitional architecture, right? We're going to talk a little bit about the architecture and how we think about this in more detail here shortly, but it does provide that way to bridge the gap.
So many leaders are just not sure. How do I get started? What do I do? I do. I just go out and get. You know, access to open the eye and in and of itself, and LLM is not enough, you know, there's this notion of hallucinization. So anyways, no, I think it's good feedback. And it's probably a key takeaway for leaders to understand that you need to pivot here, but you need to across the gap.
You don't need to jump all in and start removing other things and capabilities. Absolutely. Absolutely. I think this is one of those classic, you know, you, you get on the path and you start iterating and by being on the path, you start learning a little bit and, and then, and you, you know, you just, you just, you just go up that maturity curve.
You know what we've talked, you know, it's been about 20 minutes. We've talked, uh, you know, um, good conversation, a little bit of theory. Why don't we show, if you're, if you're ready, why don't we, why don't we show the audience, you know, an example, um, give them a little demo of, uh, AI, right? It'll just help ground some of this stuff.
I would love to. And hopefully you can see my screen all good. I'm just going to turn my video off just to make sure that we've got a good fluid experience here in the demo. But what you what you're seeing here is we've actually built a working AI assistant and this was built using rag. We're going to talk about what a rag is and a little bit more detail here as we go forward, but it uses rag, and then that's coupled with some of the open AI technology for now, though.
Let me just walk you through a quick demo of the print escape AI assistant. So for today's discussion, we're really focused on the business functionality of the app. But before we jump in, I just want to highlight a few features at a high level. Um, we've got multiple functions here. Um, we include both a chat and a QA interface, and I'll show you the difference between those and how they work here shortly.
Essentially, this allows users to explore, you know, the various options to help evaluate. The trustworthiness, if you will, or the responses from the system, the system with actual real citations for your data that's really coming out of your enterprise, not just you know what some folks have thought about to put into the LLM.
Um, and it also allows you to track that source content, et cetera. So for, for the, to the first example here, let's just ask the AI data assistant, a question from a data governance perspective, uh, say I'm a new employee and I'm working with the finance team, I'd like to know. What are some of the common terms in in the financial space for the business on in that case, it really just couldn't be easier.
I'm going to ask the assistant. I'm going to say what are the actual accounting terms. So let's just put that in here. And for brevity, I'm just going to copy and paste. And then let's just go ahead and ask that assistant. So the the process is that it's going to go into and we'll we'll cover some of this in in the architecture.
But essentially, the assistant is going to go out and look at real information coming from the business. And then, as I mentioned, what it's doing here, you can start to see that it's giving you a sample of what those associated terms are, right? And, uh, these, the selection of terms is really enough to get me started.
It's not complete, but you can see that for each one of these, there's, there's actually a citation, uh, reference. And if I wanted to know more, I'm able to simply click the provided link, uh, to, to the information from the assistant so I can get more information and, and go in and, and figure out what that looks like.
So. It's it's anchored in real information that's actually coming out of the business, which is good. And then the LLM has has helped it to do that. Now, if we take a look at some of the top questions, let's just clear this here. And, um, you know what? What are questions that are asked around the business on a regular basis?
Um, you can see that the data system has actually surfaced some of these top questions here in these examples. And you know, I'm curious to know just just what the role of a of a business steward is. So I'm simply going to going to click that example that's been presented by the data assistant right here.
But before I do that, there's there's some additional capability that we've surfaced here. And this, this really kind of goes to the heart of tuning the assistant, getting it smart, getting it to learn your business, right? Um, that the A. I. Aspect of it. Um, and What I want to do is I want to demonstrate how we can we can get the assistant to to prompt us with some follow up questions after we we answer this one.
And that's going to be really based upon how other people in the team are interacting with the model. So I'm going to open up the developer settings here. And then down here. These are some configuration pieces. I'm just going to say I want the tool to suggest some follow up questions and then I'm just going to go ahead and close that.
OK, so let's go ahead and ask the example question from the from the chat bot. OK, and here you can see that it's actually. Pretty, uh, intelligent in that it understands you're trying to understand more about a role. So it gives us a little high level overview of what that is, uh, again, it's giving me some citation.
So it says this is anchored in some real information out of the business. Uh, it also gives me some some follow ups there. Um, I'm gonna click on this articles JSON link, and this is gonna kind of drive into that. So this is data in a JSO format that was directly extracted from a data catalog. What, why that's important is we're showing that in fact the, the chat bot is, we train it, is taking real information from the catalog and then if we, if we take a look at how that information is being structured and formatted from a.
Natural language process. We get a pretty good example of, you know what that looks like, um, inside the the model itself. But the cool thing about that, too. And I don't want to get too technical in this, but I just want to share it with you to let you know that, um, you know, we've we've we're trying to show that there's possible approaches to prep that data and to prompt and how to build those prompts.
And then ultimately, Yeah, absolutely. How do you orchestrate the interaction between both the model, OpenAI or another LLM of your choice, and then the retriever, which in this case, we are built on Azure AI Search. So that's actually doing the retrieval work for us. Um, and. Again, this is probably a little bit more technical than we wanted to be in terms of this demo for the A.
I. Assistant. It's important to demonstrate that you know how we or your folks can help you to actually, um, further tune the A. I. Assistant. Here's just an example of what the thought process is. You can go in and you can build these. These are this is the actual detail of how you build and manage those prompts.
Okay, So let's let's do this. Let's go ahead and, um, we're gonna I want to show an example of how to directly query information out of your business. And in this case, um, we're gonna ask a couple of corporate data questions, and I want to make sure again that I'm getting content directly from the content we've uploaded from our business.
So now the data system is going to provide us actual data answers from various sources in the training model. And in this case, let's ask this, and again, I'm just going to type this in, um, make sure we're here. Um, let's say, what are my, and we're going to say business domains. There we go. And let's go ahead and ask that question.
What we get back here is a list of functional domains in the business, and these are associated with a single citation. Again, back to the citation, all of this is grounded in, in actual data, the source. And in this case, it comes from a business domain extract out of the catalog that was then uploaded as an Excel file.
Now, in many cases, the, the different ways that you Import and load that information will have some some impact by the technologies that you're using in your business, but it's always easy to teach the model how to understand those particular things. So in this case, um, you know, we've we've asked the question.
It's a here's the things in our fund company called Contoso, and these are the fundamental business capabilities of the business domains. Okay, so pretty quick. Uh, just wanted to go through and show you how the AI tool is working. The AI data assistant. And, um, I think at some point this demo hopefully helps you to anchor what your next generation workflows might look like using an AI assistant.
Yeah, it's great. Thank you, Chris. Appreciate it. I'll add a couple of comments on here. Um, you know, what we're showing is kind of a, um, a template right for a data system. As I said earlier, like, with the roles, what we would typically do in an engagement is we help you work through that matrix. I showed, you know, what roles you want to implement first at what level of detail.
And then we help identify what internal enterprise content, including content, the catalog is going to come in. Right. So we'll help, help bring that in. And then, um, we can tailor this, uh, this UI, right. To fit, fit what makes sense. Um, so that's the general overall theme I had just to share a couple, a couple examples.
I had, um, I had a, uh, a CIO, um, a couple of weeks ago who was really frustrated cause he's going to all these meetings and he said, you know, his, his leaders, right. So it's people pretty high in the organization. Are, um, presenting, uh, information and graphs and charts and inconsistent ways. And so he goes from meeting to meeting to meeting.
He's trying to understand cross functionally how the business is working and he's seeing different representations of data. And so he's frustrated because. They, as a group in every meeting, they have to re figure out what they're all talking about. So he said, can we create some sort of a style guide?
Because one of the things I'm doing is working, working with him on creating a data, uh, data literacy, um, and data learning program. But he said, can we include style guides, right? Suggestions for how people should be representing data, right? I really would like to get everyone on the same page. And, you know, there's a perfect example of something for an instructional type data system that you'd bake right into the data system and then people can can ask the data system what's the best way for me to present, you know, certain type of data and right to have it have to tell you that.
So it doesn't have to be just, um. details about data or analytics, but can also be learning, um, in educational materials. So that, that was one. And then you can actually extend that example to go one step further and say, if the data assistant can actually do some of the analysis. And it already knows what the standards for presenting are, then it can do the analysis and put that all in the same visualization, all in one fell swoop.
Right. So, um, you can actually, you can actually one up that another example I'll give where I think a data assistant would be a great, uh, would have been a great lift. This was before the days of, of Jenna, uh, uh, generative AI, but I was hired by an insurance company and on the East coast to come in, they were doing a major shift of their policy.
And claims, um, applications, right? So that's massive. That's the core of an enterprise of a insurance company. And they're trying to in parallel move their entire analytics and reporting and BI platform. Bad decision. You should always do those one after the other, in my opinion. But anyway, uh, it was a mess and they hired me to come in and sort of straighten that out.
One of the main things I found is that as they migrated data over a multi year migration, people didn't know where data sat anymore. They literally didn't know who are the experts. On specific types of data and was an old system new system operational system analytics system in flight somewhere in between It was causing huge headaches, wasted time, email trails galore every day by every function in the business, trying to figure out who the experts are.
Where's my cheese, where'd the data go? And so it would have been a great, great use of a data assistant to know all that and to bring, you know, uh, order insanity to the, to the madness. Um, so, you know, just something else I thought of the other day, which would have been a great, great application. Yeah, I think a lot of people are facing that situation is more and more data and it's supporting metadata and business systems.
Um, all these micro, uh, architectures come into play. There's a lot of complexity that's being added to the organization and As teams try to reduce or simplify that complexity, Invert, inadvertently, they, they usually continue to just add additional complexity, right, till they cross the gap. So, yeah, that's why I think, you know, at some point, if you can start to focus on just what's, give me the outcome.
Right. I don't know what and how to use that data, which I think is really the early the early perspective for, you know, catalogs and what they were intending to do. But, you know, not with standing adoption. That's sometimes that that's just hasn't been realized. That value. I want to ask you to say a few words about the architecture before before you do that, just to give people a glimpse, right?
Because the purpose of this webinar. As we said in the, in the invitation is really more for data leaders, but we want to do, we do want to give people like a glimpse into our philosophy and how we're approaching this from an architectural perspective, because I know most of what's out there in, uh, is, is talking about techniques for doing.
You know, for doing this. Right. And so we'll, we'll give you a glimpse into our philosophy on that. Before I do that, I just want to mention one other thing. Like we're looking at this UI classic, you know, chat GPT style, you know, chat interface doesn't have to be this way though. I went out last night because I read an article about it's, it's a little bit cheesy, but know me, know me.
ai. Maybe some of you know it, maybe you don't, I didn't know it. I saw an article last night and it's an AI, um, you know, service for people who I guess are lonely, right. To create a a mock human, right? So they can have a relationship on an ongoing basis It's got a long memory It knows you and has the interesting thing to me as it said it can also be a mentor on specific topics And I thought ah, okay, that's interesting to me, right and not the relationship stuff Um, so I went out and tried it so i'd say go ahead But that's kind of just this thing that doesn't even have this chatbot interface.
It's more of like Just a, you know, this open text conversation sort of, uh, you know, with someone else, right? Um, and it remembers everything you said for a long time, unlike chat GPT and other, most things that are out there only remember for the session you're in, and then they sort of forget context after that.
So that's interesting. And that's a whole nother approach. You can go with the data assistant, which is a much more personable, personal, um, and, and even get out of this interface, right? Yep. Yeah, I think it it's important to remember that in this case, all we're doing is we're using a textual er, you know, typing mechanism as interface.
But this is easily converted. And we're actually we have some of that capability. We won't get to it here today, but we have the capability to make this a real natural language where we're using voice based tools. And then lastly, and I want to jump into the architecture, uh, yeah. If you do get lonely, you have my number.
If you don't do that, don't do that. No, I'm open for, you know, just chatting or any, you know, I didn't want to leave you that opening. All right. Tell them a little bit about the architecture before we get too silly. Absolutely. So let's just jump over to the Prentice Gates site here. And we're actually, uh, we've got a number of this, uh, these aspects and this information available to you.
So as you get to the site, if you, If you haven't yet, get out to the site, take a look at what we're building and how we're doing it. But here's a link directly here. And again, it's going to be available for everybody. It's, it's like, my screen didn't change yet. I'm still looking at your, uh, let me share that.
There we go. There we go. Okay. Yeah. Wix 16, Chris, nothing. Um, here is the, the AI assistance, uh, page. And in this, this sense, we've got the architecture kind of outlined here. Now it's, it's at a pretty high level. But essentially, um, in this particular demo that you saw today, we have, we have other different what we call ragpacks coming.
Um, there's, there's two core pieces, and the first is this notion of, you know, getting a platform to be able to build and upload your information. We started initially, um, With the Microsoft platform, and we did so because we know that there's a lot of anxiety and concern when it comes to security and, um, you know, sharing your information.
So in in many of the solutions that are hitting the market right now, there's a lot of different ways to do this, and it uses a lot of different moving parts. Microsoft is actually making significant investments, and they're starting to push all in there. So in this particular tool that I just shared with you today.
We're using these core components. The Azure AI search. Uh, there's an Azure open AI integration, which will help you to get out to whatever your LLM is right now. It's we're using open AI, but you can use different things. Um, and then you can always go into some different tools. We're not doing that right now, but we have the ability to do that.
So. From a platform perspective, the primary architecture is probably already there in your business. If you're a Microsoft customer with using this AI data assistant, you're going to be all set. It's and we'll help you to configure that and let you know what you need to do there. Having said that, this is really where the next generation of generative AI is going, and this is this notion of rags, which are relational generative, um, tools that associate Real information and help you to build these prompts.
This augmented data builds a prompt then and goes back into this engine. Um, it's I don't want to get too technical, but the point is, is that there's an aspect of this a data assistant. Where we'll get information directly from the catalog. We've shown you some examples of that, uh, things like lineage and models and, and, uh, pdfs coming from all sorts of different aspects.
But the point is, is this is all your data coming from your business that has already been curated. So it's leveraging. The context, and it's teaching the model context about your business, and that's really what this early architecture for this particular assistant looks like we have other assistant to technologies and architectures that we're developing right now, um, some that leverage, uh, the Google platform, the AWS platform, and then, you know, a broader tool sets like Lang chain, etc.
So we've got a number of things in the works. This is just kind of our first release. Because we think this is probably the easiest to get up and running. We literally can get this up and running anywhere from a P. O. C. In a couple weeks to actually a full implementation in, you know, probably just under 90 days.
So that's what the architecture looks like. And pretty easy to implement comments on this, Chris. Um, I think I've stopped already. Sorry. That's okay. It's fine. Um, a couple comments. One is Our experience, so look, you can go out to chat. You can go out to a lot of different services and you can even, you know, copilot inside Microsoft.
Now it can scan your, um, it can scan your drive for you. You're one drive and right. You can read your files, right? So in 1 sense, this retrieval augmented generation generative capability, this rag capability is quite easy, right? You can just point at things and pull it in. But what our experience has shown is at scale, it's not easy to get right, right?
Uh, that that's where I think you, you need someone with expertise of knowing how to ramp it up and make it more, make it more accurate and, and give a higher quality response. So that, that's one comment. Now there's lots and lots of people out there, including us who are working really, really hard on that and are gaining lots and lots of experience can bring that to bear, but there's lots and lots of people.
I think where Chris and I. understand our secret sauce to be it's in our deep, um, enterprise data domain expertise. And that box at the top with all those subjects. In fact, Chris, one of your first questions to me about subject matter and domain and what, what do we put in this thing? It's thinking about what you should put into it, playing what roles.
And that's where Chris and I were really focused on this idea. He used the terminology rag pack, right? But we're trying to, you know, Communicate that we're going to be packaging our enterprise data expertise in these domain areas. And then, you know, and then and then feeding into an architecture. So will we be experts with, um, um, you know, with with the architecture?
Absolutely. We already are. We're way ahead of a lot of other people, uh, in our learning curve, and we can cut down your time significantly. Um, but also long term, our secret sauce, I think, is going to be in the rag packs and the and the domain expertise around around data. Um, so anyway, that's just just one comment there.
Um, I'm also going to, um, I see Samantha's back. So that means the old clock on the wall must be getting to our Q and a time. So, um, Samantha, a few more comments and then, uh, you know, um, we'll cut over to the to the questions. Um, Chris, I know that a lot of people get concerned about, um, you know, IP, right?
Intellectual property. And there's been a lot written about white. When you type things in the chat bot, is it scraping that and putting it into a public domain, LLM, and then suddenly the world can see it when you put your, your files in through this rag architecture, right? Is it exposed to the world? Is it.
Right. I mean, so, you know, can you talk a little bit about that? Just maybe allay any concerns people have? I think it's important because if you don't do it well, those concerns are valid. They happen and they're happening a lot, right? So, uh, what we're finding is that some of these, these larger language models are actually starting to feed off of, you know, private data and information.
And that's a huge concern. And so this is one of these areas in AI that everybody. As you know, we see a lot of this, this discussion about, you know, private privacy, protecting our information, et cetera. Um, every day, the approach that we've taken. And one of the reasons that we've used that Microsoft platform to start with is what we want to do is we want to focus on the enterprises that have a very specific platform, cloud based platform that's already working.
So in case of the Microsoft content, Yeah. You're getting all of your content directly from your private cloud. And what we're doing is we're creating the storage and the search indexes. And this is, you know, not to get too technical, but we upload stuff into your cloud and we've vectorized that, which is a 10 phrase for chunking it into little pieces to teach the model, how to get smart.
You know, some machine learning. All of that lives in your own environment. And then what happens is it's, uh, it's what we would call a passive prompt. And all the LLM is really doing is helping us with natural language. It's not helping us with data. It's helping us To be conversational for the assistant.
So it's really, you know, there's a couple of approaches like I mentioned. Ours is that we build in the security right away to make sure that it's not an issue and who wants to worry about that, right? I'm going to go out and I'm going to take advantage of A. I. And then, you know, the board's going to come and beat me up because now I've got, you know, public for my information is out in the public.
No, no, we want to make sure that that's off the table. Hopefully that's helpful. Yeah. Thank you, Chris. Appreciate that. So, Sam, before I turn it over to you and see if we have any questions, um, You know, I just want to round this out by saying, you know, so print a skate advisors. Um, so what are we doing with this, this new practice area?
Well, a, we're trying to, you know, bring, um, premeditated packaged bronze, silver, gold engagements. Um, to customers so they don't have to think really hard about what's the right way to get on the path in the scope and you can start, you know, with just a very elementary, get a pilot sort of up and running, get feedback internally.
Right? So we've got a very premeditated, easy, you know, sort of packaged way. Secondly, um, we're using, uh, what we're learning and our experience. We're coming in with a very prescriptive approach to doing that, right. Which is meant to be very, very light lift for your people internally. We know every organization, everyone's flat out.
And the last thing you, you have time for us to send your people out, uh, on an experimental project, all that I don't consider these experimental, but anyway, to make it very step by step and very light lift for your people. Um, and then, um, and then third, it's really to, uh, to, to demonstrate that, um, especially around assets you already have, like catalogs, it's how we can, uh, have our prebuilt premeditated rag packs that already know how to pull data out of those.
Put them into this natural language that Chris was demonstrating and have a very short time to value, right? So you can, you can see for yourselves how adoption can be viral in the enterprise, right? So that's really the three things we're focused on now. I would say one more future ish, but really not that far away thing I will just mention is, you know, um, You know, what we're already seeing in, in the marketplace is the coming together of multiple data assistants or agents, as some people are calling them in virtual teams.
And so this is one of those sort of sounds like rocket science things, but I would encourage any, everyone to go out and look at crew AI crew AI is a. new open source project and it allows you to create multiple what we're calling data assistants who work together. They have their own persona, their own roles, their own goal, and they can autonomously work together to do problem solving.
And so I think where we're really going, for those of you familiar with my book that I wrote last year called, you know, the goal is autonomous data governance were really Much faster than I even thought was going to be possible. We're there and we're that is becoming very capable, very possible. So again, I was challenging all of you to think about a data system is hiring a new employee.
I would go further to say you could think about data assistance is hiring a team of employees that actually work together. Uh, for, um, for specific purposes and specific goals. So I encourage you to all take a look at that in your reading and you're studying and how you're thinking about the roadmap of where your data data programs are going to go inside your enterprise.
I'd like to add one more thing to that, if I may, we, we talked about, we joked a little bit at the top of the hour about, um, you know, the number of years that we've, we've been working in this field. And I think that's kind of the pivot point for us right now is that a lot of the work in our focus area is really about bringing data practice and maturation around process and the people.
to this notion of artificial intelligence and these assistants as well. So in, in our materials, in blogs, in, in, in podcasts that John and I will do and additional stuff, we're really going to pivot hard into the focus of what does this mean for, you know, think about that typical demo wheel or that typical data management perspective.
Um, and then while Well, we're certainly helping with the technical aspects of it. I think we're certainly interested in helping data leaders navigate those landscapes and really helping with some early thinking of what do you think the impacts are of this technology on, say, a governance program or a data quality program or any number of things, you know, business process, et cetera.
So it's not just about tooling. Like, like always, right? It's always about understanding the, the capability, the process and the people and how the people intersect and interact with these things. And, and that's going to be a big focus area for us in the, in the program as well. Yeah. A hundred percent. So, um, Sam, do we have any, um, questions that anyone wants to toss at us?
Yes. Yeah. Thank you, John. We do. Um, I'd like to start off with first, uh, a couple of common questions from current clients. So the first one, yeah, it's going to be, sounds like it might take a long time, a boatload of consultants and a lot of customer time. Does it, can you talk about how long it can take to implement and have something of tangible value that customers can start using?
All right. Uh, uh, good, good question. So, you know, I think what, uh, what, what Chris and I have is a bronze silver gold package. So we've again, tried to premeditate that. You know a bronze package a month and that's going to take you know, we're going to have one consultant on that um, and then and then you go up to Two months and then three months for the various packages And obviously you're going to have varying degrees of depth in terms of content and capability again Go back to the very first matrix I showed, you know in terms of the role and the depth there Uh, based on those packages and, and the most you would ever have for like a gold, uh, sort of implementation package would, would be two consultants, right?
For, for that period of time. So from a, in a grand scheme of things, it's a very, um, affordable, um, very, uh, again, in the grand scheme of things, very quick, uh, sort of implementation, uh, process. So, um, you know, but then we all know in the consulting world, mileage varies. So we, we always love to have a conversation about your scope, your objectives, make sure that they're lined up with the packages that we've already premeditated, but, um, and then adjust accordingly, but it does not have to be a 15 person billing for six months type of at all engagement, right?
In fact, uh, with all the modern tools that are out there today and the ones that are coming on the market every day. Every day, it seems like or every week like, uh, that almost seems ludicrous at that at this point, right? So it doesn't have to be that way. Um, Chris, anything to add to that? No, I think that's that's right.
I mean, you always have the opportunity to, you know, over index and overdo things organizationally. We certainly don't don't think that way. Um, but I think one of the values that we bring to is, you know, we're gonna we're gonna let you know when you are over indexing. Right. And how to right size the approach and the good news is, is literally some of the stuff that we've shown you here today.
Um, this, this goes very quickly. I think like most, uh, implementations, it's really again, the people aspect of it. It's change management within the organization. So to the extent that you can streamline that, um, we're certainly not going to put any, any heavy pull on that. It's going to, it can go very quickly.
Thanks, guys. Um, second and last current clients. Common question. What are some practical things that data leaders could do right now to move forward with a I data assistance? Yeah, it's good. Good question as well. Um, so, okay, so I'm going to read that as a very pragmatic question, you know, like, okay, great.
Some of this sounds theoretical. Some sounds more concrete. And, you know, Chris demonstrated, you know, concrete things that are, you know, technologies that are being used today. So, so what do you do about all that? I guess my, my advice would be to, I've said it a couple of times, get on the path. What does, what does that mean?
That means I think, um, I think you, this is one of those things you can't unsee it. Right. And we, and we've all seen it. Yeah. Gen AI is here. It's not going away. Right. I don't think the head in the sand is the right, is the right, is the right strategy, uh, career limiting strategy, I think. So I think let's get on the path.
So I think at a bare minimum, it's, um, decide to do an instructional or a librarian style, you know, um, data assistant, stand it up for a month, feed it with some of your own through a rag model, like Chris demonstrated with your own knowledge and content. If you have a catalog, you know, we can help you connect to it really quickly and start piping some of that data in and then we'll help you define a scope of an audience to expose it to, to get feedback, right?
Doesn't have to be the entire enterprise can be, but, but get feedback and then start that, that, that, that iterative process. You'll learn so much, um, from that first iteration that then it will, and we'll help you, but it will inform, you know, what comes next. Right. So that would be my, um, my advice. It doesn't have to be super expensive.
In fact, it's not super expensive to do that. Um, and so, you know, why not? Right. I think you, you, and, and also like all of the data leaders that I'm consulting with right now, like I, I said earlier, the C suites giving, you know, applying pressure, uh, that's true with every one of the data leaders that are my clients currently, right.
They're, they're all being asked, what are you doing in AI space? Um, and so I think it's also, uh, in some sense, it's defensive move as well to demonstrate that you're doing something. I don't know, Chris, anything. I think one key point that would be important is for the very same reasons that we have challenges with adoption in data catalogs or other data related or technology tools.
Um, to the extent that executives can bring their Um, to Energy to bear on knocking down those silos and making that information and access to that information for the team that's going to do the implementation easier and better to get to right. In other words, this is my data. I don't want to share it.
Take that off the plate, right? Make sure that now, um, an AI team has accessibility to to build that architecture in and That's gonna go a really, really long way. It's classic, right? Data silos kill you in a lot of different ways. They will kill your your migration to A. I as well. If you don't manage that effectively, that's exactly what the leader should be.
That's a great point. So, Sam, I know you want to take us into a wrap up, but I want to make a few comments here at the end again. I want to thank Chris for his participation, his hard work on the demo and really, as you all saw, I mean, We're, we're as much partners in this as, as moderator and, and speaker.
So thank you very much, Chris, for your participation. Sam, for all the logistical help. And thank you to all of our, um, audience for attending and your interest in all this. I think, you know, Kerniscade advisors, we, we are who you see, uh, we're, we're real people. We've been in the data space for, for decades.
Um, we, uh, we think that we're very transparent and, and that's our goal to be very transparent and Uh, call it like we see it and work with you. And so, uh, just trying to stay down to earth. And so this is one of those topics can get away from you in a hurry. There's a lot of hyperbole out there and a lot of sort of bad information.
Um, we encourage you to reach out to us and talk to us, have a conversation. We'll, we'll, we'll, you know, we'll, we'll tell you like we see it and, uh, help you the best we possibly can and try to keep it real and, uh, and, and realistic, um, you know, both from a technology perspective and what's possible and capable and.
And also from a strategy perspective and what's, what's the right strategy for you within your organization. So, uh, so just encourage anyone who, um, needs that type of conversation, you know, to reach out to us. We'd love to talk to you about it. So, um, Sam, do you want to take us, do you have any wrap up for us?
Absolutely. I think you covered most of it. Um, just wanted to mention any questions that we were unable to answer due to time. Uh, we will be sure to reach out via email, um, and follow up with you with the answer. Um, thank you everyone for your time and uh, feel free to visit the website and subscribe to stay in the know of the latest happenings with ApprenticeGate Advisors.
Are you going to share this recording out? So then folks could just go to the website too. If you want to share it near your organization, please come to the website and, uh, Sam will get us all set up to do that on the website and the email. Yes. Chris. Thanks everyone. Thanks everybody. All right. Thank you.
Bye bye.